biomodal
- Fabio Puddu
- Casper K Lumby
- Nick Harding
- David J Morley
- Jamie Scotcher
- Robert Crawford
- Jens Füllgrabe
- Walraj S Gosal
- Shirong Yu
- Dan Brudzewsky
- Jane D Hayward
- Andrada Tomoni
- Philippa Burns
- Joanna D Holbrook
- Páidí Creed
Liquid biopsy for profiling of cell free DNA (cfDNA) in blood holds huge promise to transform how we experience and manage cancer by early detection and identification of residual disease and subtype. However, a standard blood draw yields an average of only 10 ng of cfDNA, of which DNA derived from the tumour is a small minority.
Genetic and methylation data together have been shown to be more powerful for the detection of early cancer than either alone. Constrained to measuring four states of information, existing NGS-based technologies sacrifice genetic for methylation calling.
duet multiomics solution +modC is a new sequencing technology that derives all four genetic bases without ambiguity in C or T calls, plus epigenetic 5modC. The technology consists of pre-sequencing library prep and post-sequencing analysis pipeline, providing single-base resolution of genetics and epigenetics at high accuracy
State
|
Standard
sequencing protocol
|
Protocol with C→T deamination
|
1
|
A
|
A
|
2
|
C/mC/hmC
|
mC/hmC
|
3
|
G
|
G
|
4
|
T
|
C/T
|
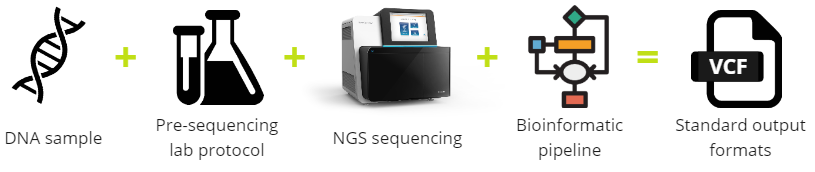
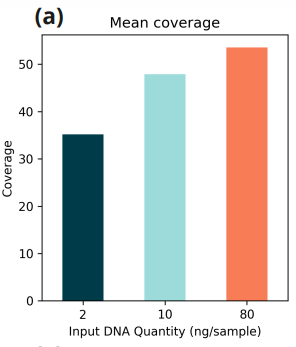
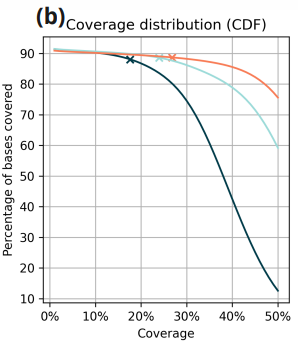
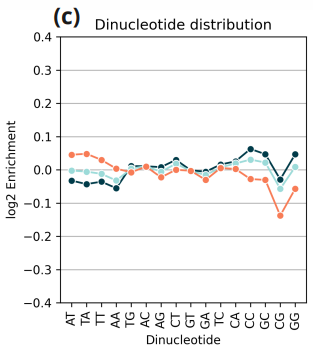
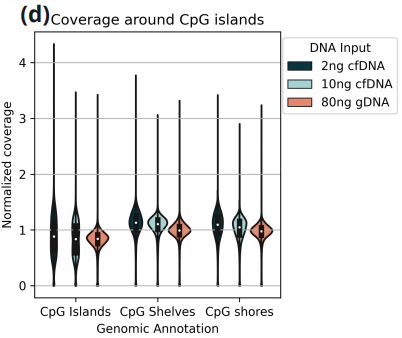
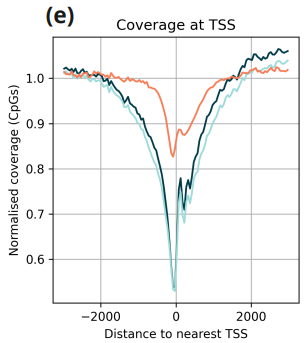
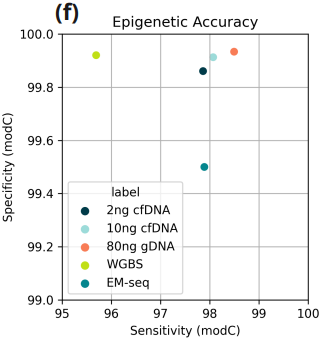
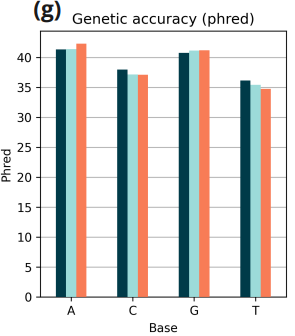
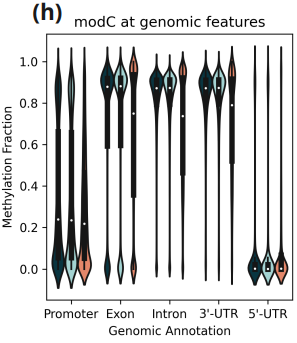
Data is presented for three duet+modC libraries: two libraries generated from 2ng and 10ng cfDNA extracted from a plasma sample taken from a single woman patient with a gastric cancer diagnosis and one library generated from 80ng of genomic DNA from the NA12878 cell-line.
- Mean genomic coverage;
- Cumulative coverage distribution. y-axis denotes the percentage of sites which achieve at least the coverage value on the x-axis. Crosses mark the position corresponding to half-mean coverage. At all inputs, approximately 88% of sites are covered at least to half the mean coverage level.
- Nucleotide bias plot showing the log2 enrichment of covered versus expected dinucleotides, calculated as log2(observed proportion / expected proportion) for each dinucleotide.
- Normalized coverage at CpG islands, shelves and shores.
- Normalised coverage in regions flanking transcription start sites.
- Sensitivity and specificity of modified Cytosine calls, computed on fully methylated and unmethylated spiked-in control DNA. Data for EM-seq and WGBS taken from (Füllgrabe and Gosal, 2023).
- Genetic accuracy computed on spiked-in lambda genome. Empirically observed phred score is > Q40 for A and G and > Q35 for C and T.
- Methylation fraction across different genomic features
Read resolution enables the suppression of sequencing errors, as these will result in pairs of bases which do not resolve. Due to redundancy in the resolution rules, we can only suppress errors at 8 of the 12 possible substitution types: A<>G substitution errors on R1 and R2 lead to A<>G and C<>T substitution errors in the resolved read.
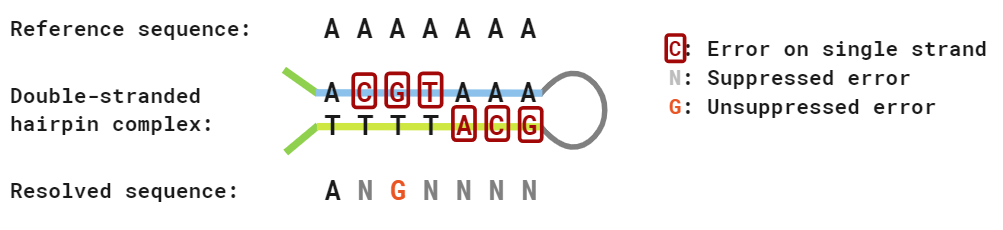
We can compute the empirical error rate at suppressed substitution types by comparing to a sample with known genotype [2]
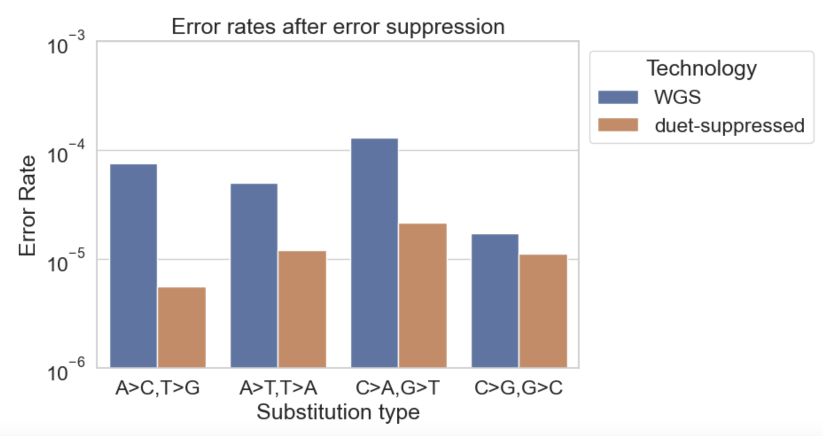
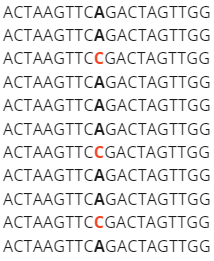
We call somatic variants depending on a cut-off value, specifying the minimum number of observations of the minor allele we must see before calling a variant.
A>C variant called if cut-off is 3
A>C variant not called if cut-off is > 3
Sequencing depth, error rate, and cut-off value determine the balance between sensitivity and false positive rate
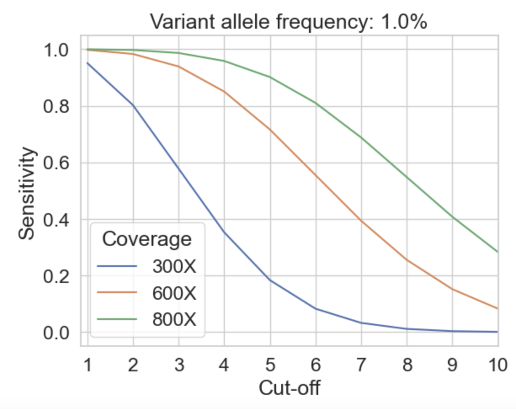
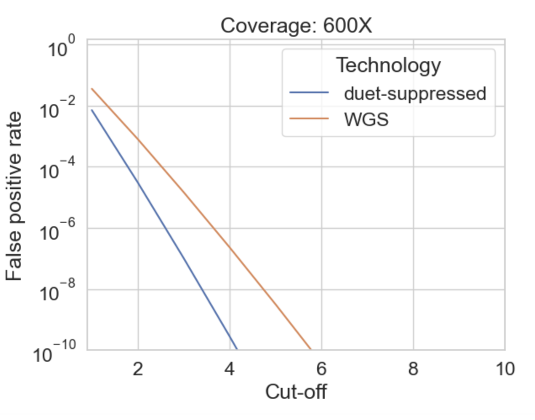
Choosing a cut-off that will give at most one false positive variant call per GB, we compute the expected sensitivity for somatic variant calling achievable using standard WGS and duet multiomics solution +modC at variants corresponding to the eight error-suppressed substitution types
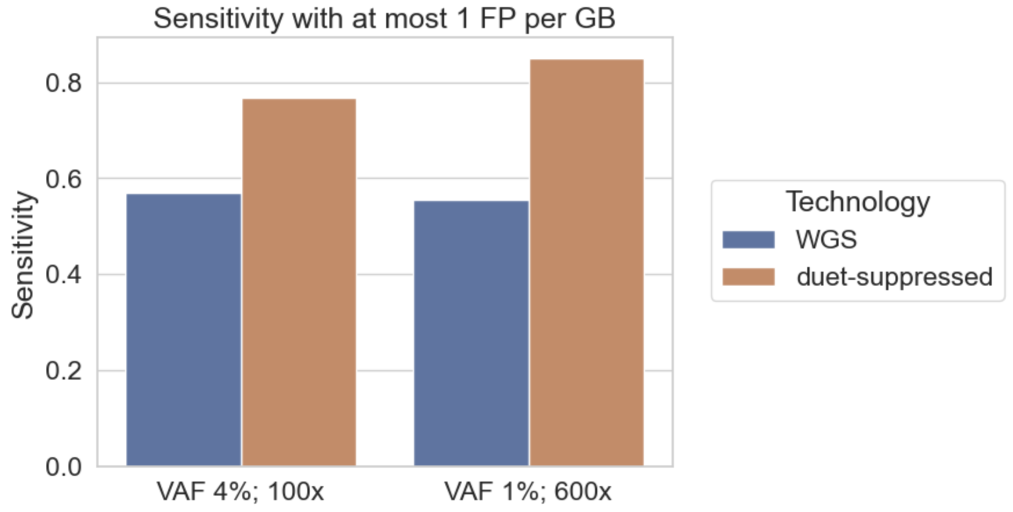
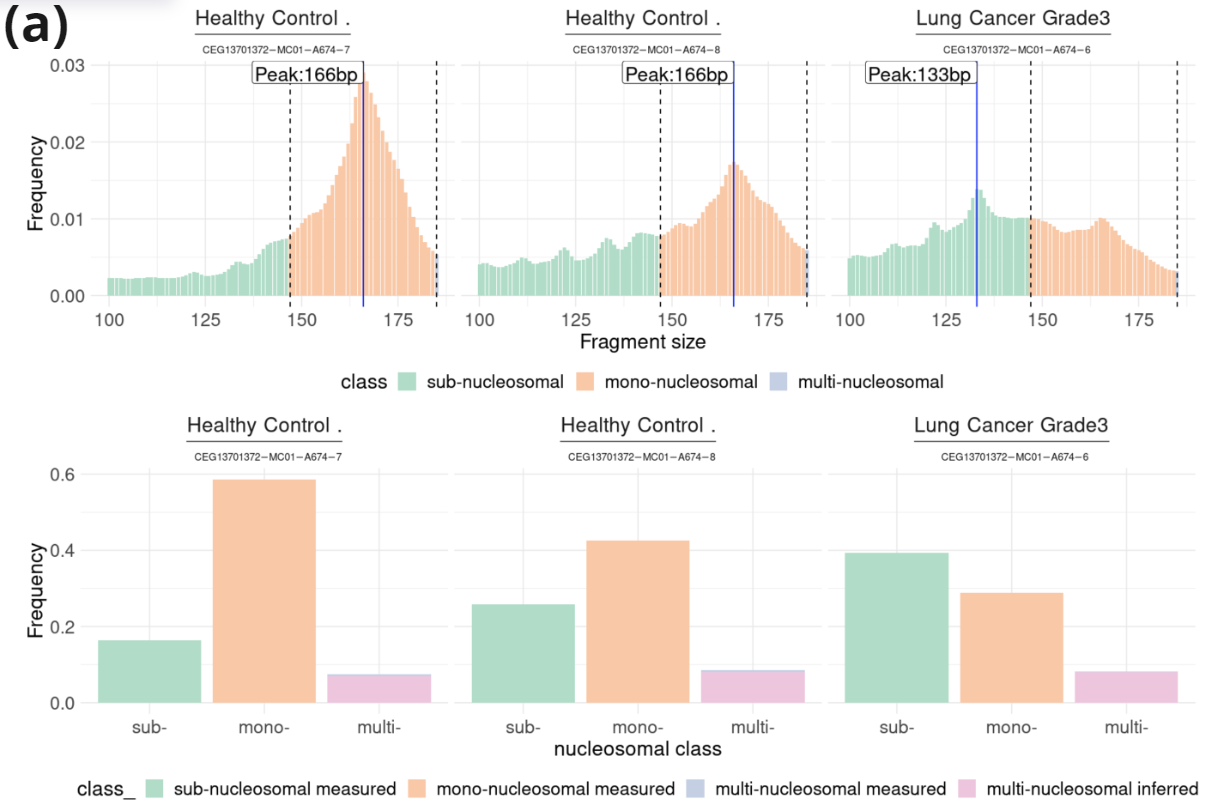
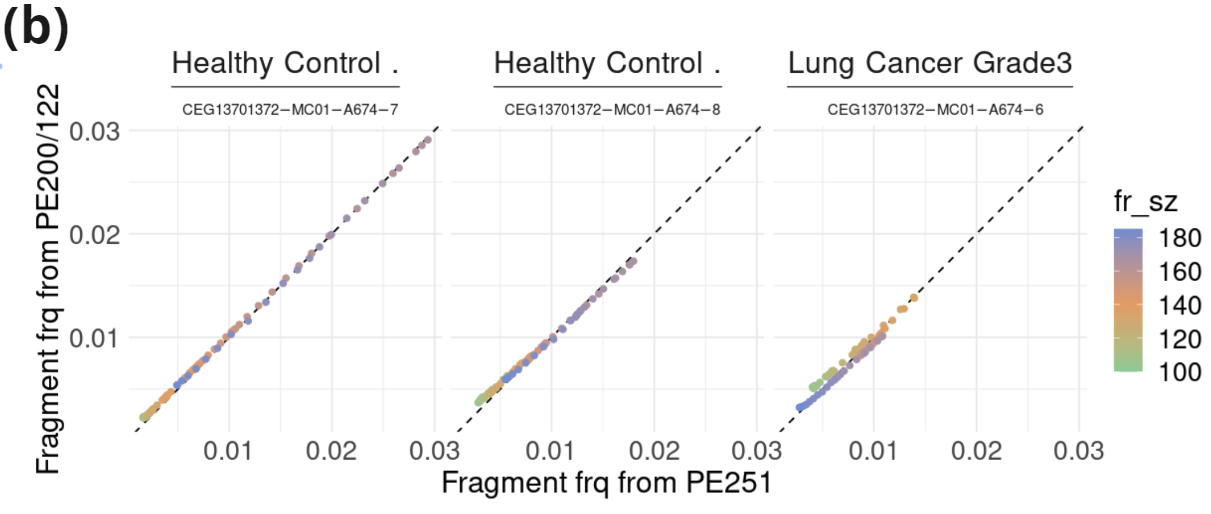
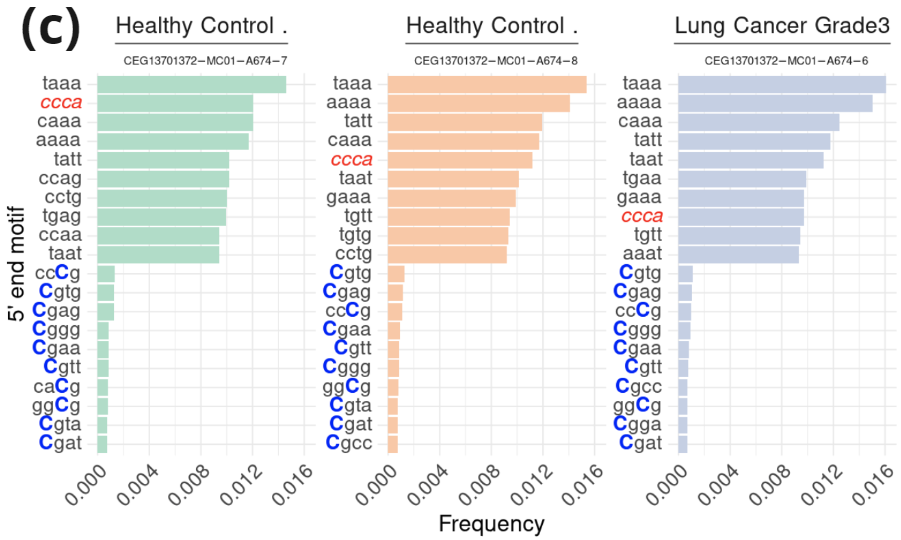
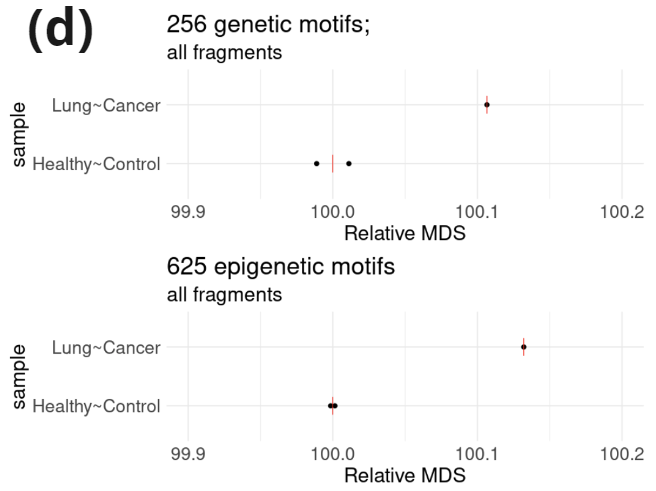

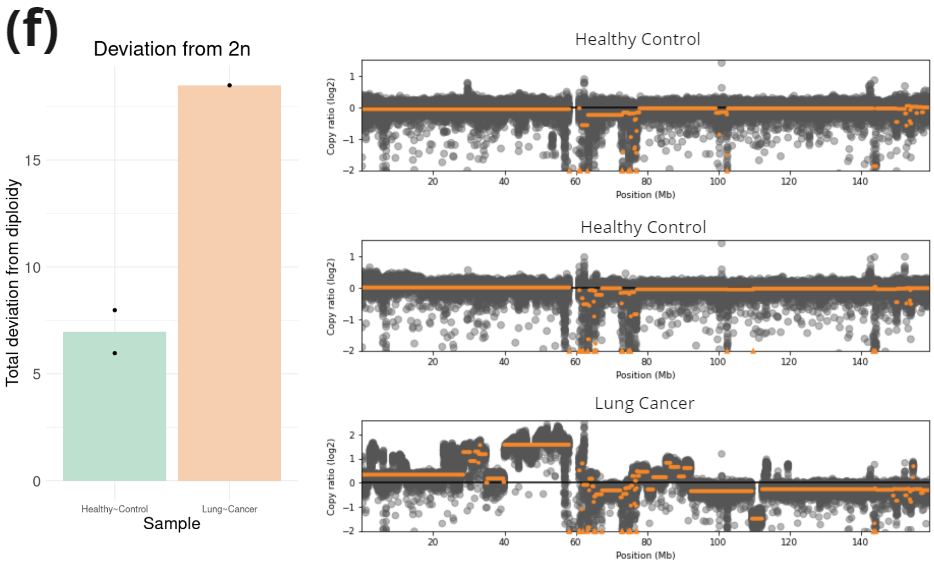
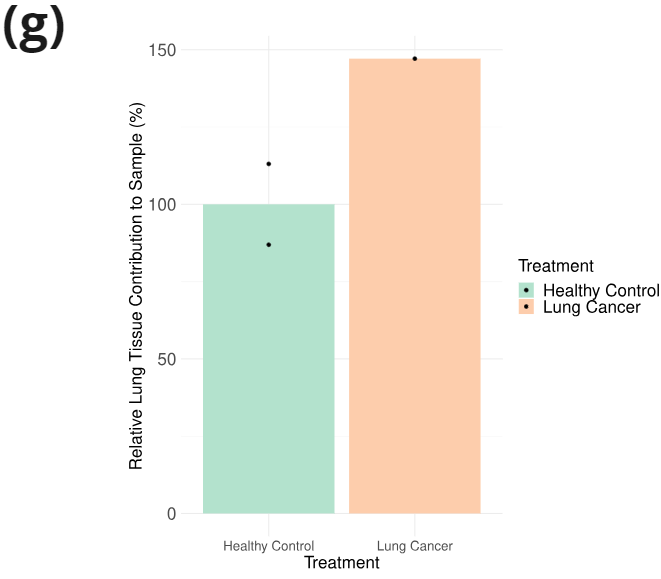
- Fragment length profile (100-185nt) and contribution of sub-, mono-, and multi-nucleosomal fragments in samples from two healthy controls and one lung cancer patient. Data was extracted from an asymmetric PE read run (PE200/122: R1=200c; R2=122c). Fragment length was inferred for fragments in which R1 contained hairpin sequence.
- Correlation between fragment length frequency (100-185nt) in independent libraries of the same samples sequenced with PE251 or asymmetric PE200/122.
- Frequency of the top 10 5′-end genetic and epigenetic (=containing modified C) motifs in mono-nucleosomal fragments in each sample.
- Relative motif diversity score [3] [ (MDS; healthy-controls=100) calculated considering the 256 4bp 4-letter motifs or the 625 4bp 5-letter motifs (including C and modC) on 5′-end motifs from mono-nucleosomal fragments.
- Read jaggedness: the Jagged Index-Unmethylated (JI-U) [4] was calculated from a symmetric PE151 run for fragments with length less than or equal to read length. Each dot represents a biological replicate (Healthy Control and Lung Cancer) or a technical replicate (genomic DNA). Two technical replicates are shown side by side.
- Copy number variation information was obtained using CNVkit [5]. Total deviation from 2n was calculated summing the average absolute deviation from diploidy for each autosome. A comparison of copy number variation across chromosome 7 is shown on the right.
- Relative lung tissue contribution to cfDNA: to obtain relative tissue contributions to each cfDNA sample, methylation deconvolution was conducted separately on each sample following the method described in [6] and using the tissue reference provided therein. Proportion of modified Cytosine levels mapped to the lung increases by 50% in the lung cancer sample relative to the health controls
We have presented data illustrating the potential of duet multiomics solution +modC for liquid biopsy. With duet multiomics solution +modC it is possible to:
- Generate high quality data from low input amounts
- Obtain more sensitive somatic variant calling through error suppression at 8 of 12 possible substitution types
- Obtain multi-modal information that can be used to discriminate cancer patients from healthy controls
We believe that this technology will help advance the field of liquid biopsy towards its promise
- Simultaneous sequencing of genetic and epigenetic bases in DNA, Füllgrabe and Gosal et al., Nature Biotechnology (2023). (duet multiomics solution technology paper)
- Extensive sequencing of seven human genomes to characterize benchmark reference materials. Zook et al. Scientific Data (2016).
- Plasma DNA End-Motif Profiling as a Fragmentomic Marker in Cancer, Pregnancy, and Transplantation. Jiang et al. Cancer Discovery (2020)
- Detection and characterization of jagged ends of double-stranded DNA in plasma. Jiang et al. Genome Research (2020)
- CNVkit: Genome-Wide Copy Number Detection and Visualization from Targeted DNA Sequencing. Talevich et al. PLOS Computational Biology (2016)
- Plasma DNA tissue mapping by genome-wide methylation sequencing for noninvasive prenatal, cancer, and transplantation assessments. Sun et al. PNAS (2015)